7. Chaos Theory for Beginners
— An Introduction —
Life finds a way
"Life finds a way"
Remember Jurassic Park? Handsome mathematician Doctor Malcom explaining to pretty Doctor Sattler why he thought it was unwise to have T-rexes and the likes romping around on an island? John Hammond, the annoying owner, promised that nothing could go wrong and that all precautions were taken to ensure the safety of visitors.
Dr. Malcom did not agree. "Life finds a way," he said.
Nature is highly complex, and the only prediction you can make is that she is unpredictable. The amazing unpredictability of nature is what Chaos Theory looks at. Why? Because instead of being boring and translucent, nature is marvelous and mysterious. And Chaos Theory has managed to somewhat capture the beauty of the unpredictable and display it in the most awesome patterns. Nature, when looked upon with the right kind of eyes, presents herself as one of the most fabulous works of art ever wrought.
What is Chaos Theory?
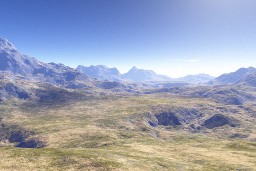
Chaos Theory is a mathematical sub-discipline that studies complex systems. Examples of these complex systems that Chaos Theory helped fathom are earth's weather system, the behavior of water boiling on a stove, migratory patterns of birds, or the spread of vegetation across a continent. Chaos is everywhere, from nature's most intimate considerations to art of any kind. Chaos-based graphics show up all the time, wherever flocks of little space ships sweep across the movie screen in highly complex ways, or awesome landscapes adorn the theater of some dramatic Oscar scene.
Complex systems are systems that contain so much motion (so many elements that move) that computers are required to calculate all the various possibilities. That is why Chaos Theory could not have emerged before the second half of the 20th century.
But there is another reason that Chaos Theory was born so recently, and that is the Quantum Mechanical Revolution and how it ended the deterministic era!
Up to the Quantum Mechanical Revolution people believed that things were directly caused by other things, that what went up had to come down, and that if only we could catch and tag every particle in the universe we could predict events from then on. Entire governments and systems of belief were (and, sadly, are still) founded on these beliefs, and when Sigmund Freud invented psychoanalysis, he headed out from the idea that malfunctions in the mind are the results of traumas suffered in the past. Regression would allow the patient to stroll down memory lane, pinpoint the sore spot and rub it away with Freud's healing techniques that were again based on linear cause and effect.
Chaos Theory however taught us that nature most often works in patterns, which are caused by the sum of many tiny pulses.
How Chaos Theory was born and why
It all started to dawn on people when in 1960 a man named Edward Lorenz created a weather-model on his computer at the Massachusetts Institute of Technology. Lorentz' weather model consisted of an extensive array of complex formulas that kicked numbers around like an old pig skin. Clouds rose and winds blew, heat scourged or cold came creeping up the breeches.
Colleagues and students marveled over the machine because it never seemed to repeat a sequence; it was really quite like the real weather. Some even hoped that Lorentz had built the ultimate weather-predictor and if the input parameters were chosen identical to those of the real weather howling outside the Maclaurin Building, it could mimic earth's atmosphere and be turned into a precise prophet.
But then one day Lorentz decided to cheat a little bit. A while earlier he had let the program run on certain parameters to generate a certain weather pattern and he wanted to take a better look at the outcome.
But instead of letting the program run from the initial settings and calculate the outcome, Lorentz decided to start half way down the sequence by inputting the values that the computer had come up with during the earlier run.
The computer that Lorentz was working with calculated the various parameters with an accuracy of six decimals. But the printout gave these numbers with a three decimal accuracy. So instead of inputting certain numbers (like wind, temperature and stuff like that) as accurate as the computer had them, Lorentz settled for approximations; 5.123456 became 5.123 (for instance). And that puny little inaccuracy appeared to amplify and cause the entire system to swing out of whack.
Exactly how important is all this? Well, in the case of weather systems, it's very important. Weather is the total behavior of all the molecules that make up earth's atmosphere. And in the previous chapters we've established that a tiny particle cannot be accurately pin-pointed, due to the Uncertainty Principle! And this is the sole reason why weather forecasts begin to be bogus around a day or two into the future. We can't get an accurate fix on the present situation, just a mere approximation, and so our ideas about the weather are doomed to fall into misalignment in a matter of hours, and completely into the nebulas of fantasy within days. Nature will not let herself be predicted.
Hold that thought (7)
The Uncertainty Principle prohibits accuracy. Therefore, the initial situation of a complex system cannot be accurately determined, and the evolution of a complex system can therefore not be accurately predicted.
Attractors
Complex systems often appear too chaotic to recognize a pattern with the naked eye. But by using certain techniques, large arrays of parameters may be abbreviated into one point in a graph. In the little rain-or-sunshine graph above, every point represents a complete condition with wind speed, rain fall, air temperature, etcetera, but by processing these numbers in a certain way they can be represented by one point. Stacking moment upon moment reveals the little graph and offers us some insight in the development of a weather system.
The first Chaos Theorists began to discover that complex systems often seem to run through some kind of cycle, even though situations are rarely exactly duplicated and repeated. Plotting many systems in simple graphs revealed that often there seems to be some kind of situation that the system tries to achieve, an equilibrium of some sort. For instance: imagine a city of 10,000 people. In order to accommodate these people, the city will spawn one supermarket, two swimming pools, a library and three churches. And for argument's sake we will assume that this setup pleases everybody and an equilibrium is achieved. But then the Ben & Jerry's company decides to open an ice cream plant on the outskirts of the town, opening jobs for 10,000 more people. The town expands rapidly to accommodate 20,000 people; one supermarket is added, two swimming pools, one library and three churches and the equilibrium is maintained. That equilibrium is called an attractor.
Now imagine that instead of adding 10,000 people to the original 10,000, 3,000 people move away from the city and 7,000 remain. The bosses of the supermarket chain calculate that a supermarket can only exist when it has 8,000 regular customers. So after a while they shut the store down and the people of the city are left without groceries. Demand rises and some other company decides to build a supermarket, hoping that a new supermarket will attract new people. And it does. But many were already in the process of moving and a new supermarket will not change their plans.
The company keeps the store running for a year and then comes to the conclusion that there are not enough customers and shut it down again. People move away. Demand rises. Someone else opens a supermarket. People move in but not enough. Store closes again. And so on.
This awful situation is also some kind of equilibrium, but a dynamic one. A dynamic kind-of-equilibrium is called a Strange Attractor. The difference between an Attractor and a Strange Attractor is that an Attractor represents a state to which a system finally settles, while a Strange Attractor represents some kind of trajectory upon which a system runs from situation to situation without ever settling down.
The discovery of Attractors was exciting and explained a lot, but the most awesome phenomenon Chaos Theory discovered was a crazy little thing called Self-Similarity. Unveiling Self-Similarity allowed people a glimpse of the magical mechanisms that shape our world, and perhaps even ourselves...
And while you wait for the next web page to load, think about this: A snowflake is an object composed of water molecules. These molecules do not have a common nerve system, DNA or a chief molecule that calls the shots. How do these molecules know where to go and hang in order to form a six pointed star? And where do they get the audacity to form a different one every time? How does one molecule in one leg of the flake know which private design the rest of the gang is cruising for, in other legs of the flake, for the tiny molecule a million miles away?
Not a clue? Go to the next chapter:
Self-Similarity →
Summary 7: Chaos Theory for Beginners; an introduction
- A tiny difference in initial parameters will result in a completely different behavior of a complex system.
- The Uncertainty Principle prohibits accuracy. Therefore, the initial situation of a complex system cannot be accurately determined, and the evolution of a complex system can therefore not be accurately predicted.
- Complex systems often seek to settle in one specific situation. This situation may be static (Attractor) or dynamic (Strange Attractor).